A team of researchers from Stanford University’s Autonomous Systems Laboratory posit that artificial intelligence (AI) could enable a form of traffic congestion pricing that could make everyone at every income level better off.
Transporation experts have spent decades searching for ways that could help reduce urban traffic jams, with one possible solution being congestion pricing. Congestion pricing would place tolls on crowded roads to help lower traffic and redistribute it elsewhere in the city. However, a top concern regarding the adoption of road pricing is that it could contribute to economic inequality, and enable wealthy users to enjoy quick travel times while low-income travelers are effectively priced out of the faster roads and bear the burden of being stuck in traffic.
However, Stanford researchers believe they have an approach that could improve economic equity while reducing congestion. According to a Stanford news release, the key idea behind the proposal is to refund tolls in a way that redistributes some of the money from rich to poor and ensures all drivers are as well off or better than they were before. Lower-income drivers would get back more money than they spend. Wealthier drivers might get some money back, but much or most of their compensation would be in the form of time they don’t waste in traffic jams.
“We can achieve both the goals of equity and of efficiency by enabling people to trade time for money,” says Devansh Jalota, a doctoral candidate at the Autonomous Systems Laboratory who carried out much of the work. “Some people can reduce their travel time by paying additional tolls, while others are compensated for taking longer routes.”
Key to this approach, researchers say, is using AI and big data to accurately predict how people in a particular area will respond to different pricing schemes. Researchers explain that every city is different, and a huge number of variables affect traffic flow, but AI makes the proposed approach realistic.
“Advances in big data and machine learning have enabled us to design better autonomous vehicles by learning patterns in the behavior of human drivers,” says Jalota. “These tools can play a similar role here in calibrating the pricing models to make our proposed schemes work.”
While researchers are confident in their proposal, they do warn that this approach will require a “tremendous amount of data and computation.” Additionally, developing a system for a city would require testing different pricing schemes, collecting data, and using machine learning tools to build specific behavioral models for how different travelers value money and time.
“If we want to deploy the whole scheme, we need to understand people’s behavior,” says Jalota. “We have a general behavioral model, but we need to learn exactly what the parameters are.”
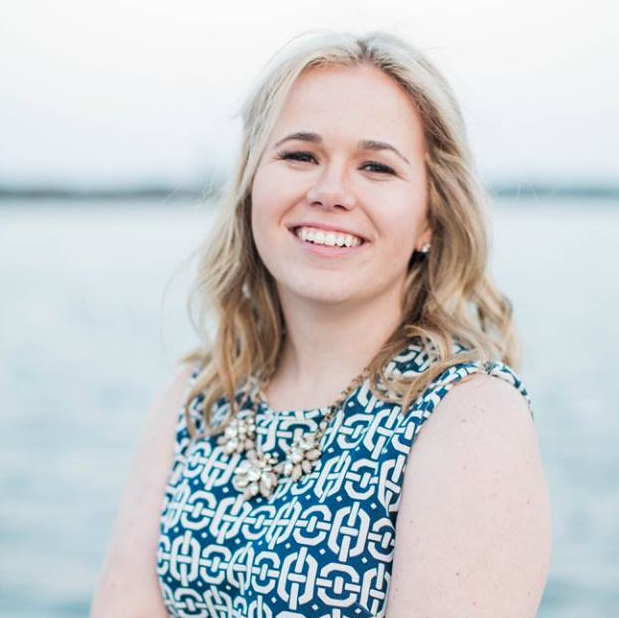